In many natural processes, random variation conforms to a
particular probability distribution known as the normal
distribution, which is the most commonly observed
probability distribution. Mathematicians de Moivre and
Laplace used this distribution in the 1700's. In the early
1800's, German mathematician and physicist Karl Gauss used
it to analyse astronomical data, and it consequently
became known as the Gaussian distribution among the
scientific community.
The shape of the normal distribution resembles that of a
bell, so it sometimes is referred to as the "bell curve",
an example of which follows:
Normal Distribution
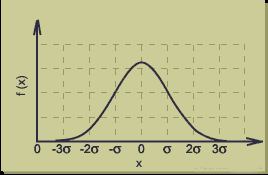
The above curve is for a data set having a mean of zero.
Bell Curve Characteristics
The bells curve has the following characteristics:
-
Symmetric
-
Unimodal
-
Extends to +/- infinity
-
Area under the curve = 1
Completely Described by Two Parameters
The normal distribution can be completely specified by two
parameters:
If the mean and standard deviation are known, then one
essentially knows as much as if one had access to every
point in the data set.
The Empirical Rule
The empirical rule is a handy quick estimate of the spread
of the data given the mean and standard deviation of a
data set that follows the normal distribution.
The empirical rule states that for a normal distribution:
-
68% of the data will fall within 1 standard deviation of
the mean
-
95% of the data will fall within 2 standard deviations of
the mean
-
Almost all (99.7%) of the data will fall within 3 standard
deviations of the mean
Note that these values are approximations. For example,
according to the normal curve probability density
function, 95% of the data will fall within 1.96 standard
deviations of the mean; 2 standard deviations is a
convenient approximation.
Normal Distribution and the Central Limit Theorem
The normal distribution is a widely observed distribution.
Furthermore, it frequently can be applied to situations in
which the data is distributed very differently. This
extended applicability is possible because of the central
limit theorem, which states that regardless of the
distribution of the population, the distribution of the
means of random samples approaches a normal distribution
for a large sample size.
Applications to Business Administration
The normal distribution has applications in many areas of
business administration. For example:
-
Modern portfolio theory commonly assumes that the returns
of a diversified asset portfolio follow a normal
distribution.
-
In operations management, process variations often are
normally distributed.
-
In human resource management, employee performance
sometimes is considered to be normally distributed.
The normal distribution often is used to describe random
variables, especially those having symmetrical, unimodal
distributions. In many cases however, the normal
distribution is only a rough approximation of the actual
distribution. For example, the physical length of a
component cannot be negative, but the normal distribution
extends indefinitely in both the positive and negative
directions. Nonetheless, the resulting errors may be
negligible or within acceptable limits, allowing one to
solve problems with sufficient accuracy by assuming a
normal distribution.

|